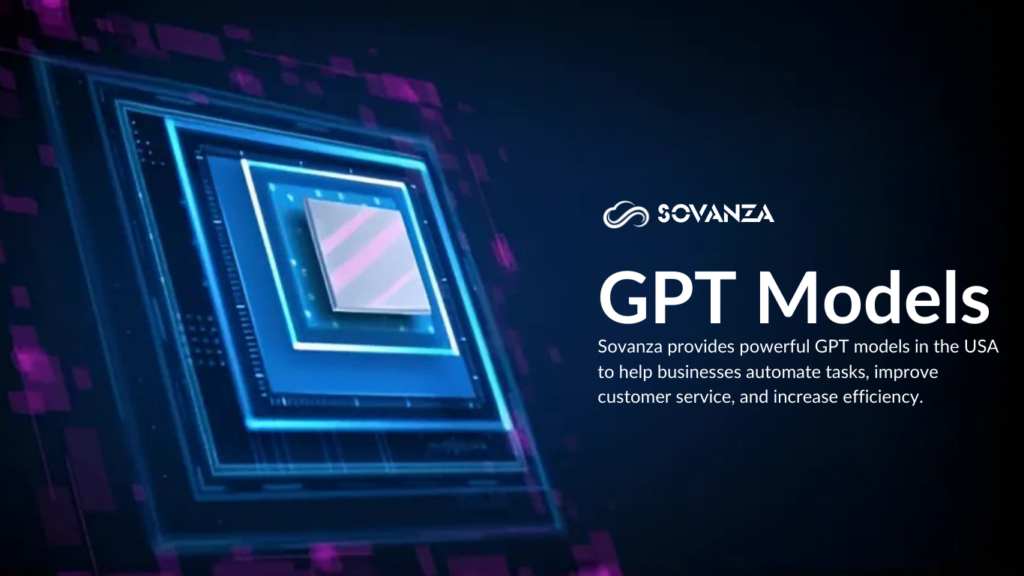
Introduction
GPT models have become an essential source of innovation across industries in the United States. These advanced AI technologies are transforming businesses’ operations by making procedures more efficient, improving consumer experiences, and automating challenging activities. GPT models, which can interpret and write human-like text, are used for everything from content production to customer assistance, generating tremendous growth and optimization prospects across healthcare, marketing, finance, and technologies.
Sovanza specializes in using the power of GPT models to drive business transformation. Our team is dedicated to providing tailored AI solutions that assist businesses in optimizing their operations, streamlining workflows, and increasing customer engagement. With an emphasis on security, scalability, and innovation, Sovanza assists US organizations in successfully integrating GPT technology into their plans, ensuring they remain competitive in an AI-driven environment.
What are GPT Models?
GPT (Generative Pretrained Transformer) models are powerful AI systems that can read and generate human-like language. After extensive data training, they can answer queries, write material, and engage in conversations. These models use a transformer architecture to understand language patterns, allowing them to produce logical, contextually accurate replies.
The most recent models, such as GPT-4.5, are much more powerful, providing superior accuracy, creativity, and contextual comprehension. Nowadays, organizations across the United States will use GPT models to develop tailored customer experiences, automate operations, and improve decision-making processes.
Applications of GPT Models in 2025
GPT models have transcended their initial use cases and are being adopted across numerous industries. Sovanza is utilizing models to enhance its offerings and improve the AI landscape in the USA:
Customer Support and Chatbots
GPT models have revolutionized customer service by powering intelligent chatbots. These bots can handle customer queries, troubleshoot problems, and provide real-time personalized recommendations. Sovanza, through its innovative solutions, helps companies implement AI-powered customer support systems that improve efficiency and customer satisfaction.
Content Generation
Content creation is one of the most interesting sectors in which models have a huge impact. Whether publishing blog articles, social media content, or even full e-books, it help organizations create large-scale written material. Sovanza uses these models to help clients in the United States create SEO-optimized, compelling content personalized to their target audiences.
Language Translation
As global business becomes more interconnected, linguistic obstacles might impede communication. GPT models can accurately translate text between languages, making them an important tool for businesses trying to develop abroad. Sovanza utilizes these AI-driven solutions to offer seamless translation services to Wyoming and the USA clients.
Healthcare and Medical Research
In the healthcare industry, GPT models handle massive volumes of medical data, aid with diagnosis, and generate research reports. Sovanza, in collaboration with healthcare professionals in Wyoming, is at the forefront of using GPT models to improve data analysis and research, resulting in better patient outcomes.
AI-Powered Code Generation
For software development, GPT models like GitHub Copilot are transforming how developers write code. These models can generate code snippets, suggest improvements, and even debug issues. Sovanza’s team of developers leverages GPT models to accelerate the software development process, ensuring faster time-to-market for solutions.
Why Sovanza Chooses GPT Models for USA-Based Solutions
At Sovanza, we are committed to delivering the best possible solutions to our clients. Here’s why we trust GPT models to power our AI-driven services in Wyoming and across the USA:
Scalable Solutions for Growing Businesses
As businesses grow, their need for scalable solutions becomes even more critical. GPT models offer exceptional scalability, allowing businesses to handle larger volumes of data and customer interactions without compromising on quality. Sovanza helps companies integrate scalable GPT-powered solutions that evolve with their needs.
Improved Efficiency and Cost Savings
It save money on operations by automating jobs like creating content, customer assistance, and data analysis. Sovanza assists organizations in implementing these solutions, allowing them to run more efficiently and focus resources where they are most needed.
Staying Competitive in an AI-Driven World
AI is no longer a luxury but a requirement for competitiveness in today’s economy. Sovanza applies models to assist organizations in the United States in achieving a competitive advantage by implementing breakthrough AI solutions that reduce processes, improve customer experiences, and drive growth.
Commitment to Innovation
Sovanza is committed to remaining ahead in the AI business. By constantly researching the latest developments in GPT technology, we ensure our clients receive the most modern solutions. We are continually refining our ways to maximize the potential of GPT models and provide outstanding value to our customers.
GPT Model Common Challenges and Sovanza’s Solutions
These are common challenges businesses face when using GPT models, and Sovanza’s tailored solutions ensure that clients can effectively overcome them.
Data Privacy and Security Concerns
It involve working with sensitive company data, which might generate privacy and security concerns. It is critical to ensure data security and compliance with privacy standards. Sovanza addresses these issues by adopting strong data encryption and adhering to data protection rules to protect customer information, providing businesses peace of mind.
Model Training and Customization
GPT models are often trained on general data, making them less effective for specific industries or tasks. Customizing these models for a company’s unique needs can be complex. Sovanza solves this challenge by offering tailored solutions that fine-tune GPT models for specific business applications, ensuring they deliver the most relevant and effective results.
Handling Complex and Nuanced Tasks
It excel in many areas but can still struggle with tasks requiring deep industry knowledge or highly specialized tasks. Sovanza addresses this by integrating domain-specific data and expert feedback into the training process, enabling GPT models to handle complex scenarios more effectively.
Cost and Resource Management
Implementing GPT models can be expensive, particularly for small and medium-sized enterprises, because they require significant computing resources and technical skills. Sovanza assists organizations in maximizing their investment by providing cost-effective AI solutions that increase the efficiency of GPT models while reducing wasteful resource consumption.
Sovanza is your Best Choice for the GPT Model?
Sovanza is the best choice for integrating GPT models into your business operations. It provides tailored solutions to fit the specific demands of businesses across the United States. With years of experience and a thorough understanding of AI technology, Sovanza ensures businesses can easily adopt and employ it to optimize processes, increase productivity, and improve customer satisfaction.
What differentiates Sovanza is our dedication to security, customization, and continuing support. We don’t just implement solutions; we collaborate with you to fine-tune models, protect data privacy, and improve performance over time. Whether you work in marketing, healthcare, or finance, Sovanza’s experience makes applying GPT models a strategic benefit for your company.
Start Your AI Journey with Sovanza
Contact us to get started with Sovanza for GPT models and unlock the power of AI to transform your business. Our team of experts will guide you through every step of the process, from implementation to optimization, ensuring that the integration of GPT models aligns perfectly with your business goals. With Sovanza’s tailored solutions, you can automate processes, improve customer experiences, and drive innovation across your operations. Let Sovanza help you stay ahead of the curve and maximize the potential of AI for your business.
Conclusion
In 2025, GPT models will be a crucial tool for enterprises in the United States, enabling innovation in a variety of industries, such as customer assistance, content development, and healthcare. These AI solutions improve operational efficiency, allowing individualized customer experiences and smarter decision-making processes. As more firms adopt and integrate GPT technology, its impact on industries will only expand, influencing the future of corporate processes in the United States.
Sovanza specializes in applying GPT models to help businesses in Wyoming and across the United States unlock the full potential of AI. Sovanza’s bespoke solutions ensure businesses can smoothly incorporate models to boost productivity, improve customer happiness, and remain competitive in an AI-driven environment. With an emphasis on security, customization, and ongoing support, Sovanza is the ideal partner to help businesses navigate the AI transformation journey.
FAQs
What are GPT models, and how do they work?
GPT models are AI systems that generate human-like text by learning language patterns. They perform tasks like answering questions, generating content, and chatting through advanced transformer architecture.
What industries can benefit from GPT models?
It benefits industries like customer service, healthcare, marketing, software development, and content creation. Sovanza uses GPT models to improve efficiency and innovation in content generation, medical research, code development, and AI-powered chatbots.
What makes Sovanza different from other AI solution providers?
Sovanza distinguishes itself by providing customized GPT solutions adapted to each business’s requirements, ensuring security, scalability, and continuing support. Our knowledge and dedication to innovation help companies stay ahead in the AI-driven market.
How does Sovanza ensure data privacy and security?
Sovanza employs robust encryption, follows data protection regulations, and offers secure, compliant AI solutions to protect sensitive business information.
How can I get started with Sovanza for GPT models?
Contact our team for a consultation to get started with Sovanza for GPT models. They will guide you through implementing and optimizing AI solutions tailored to your business needs.
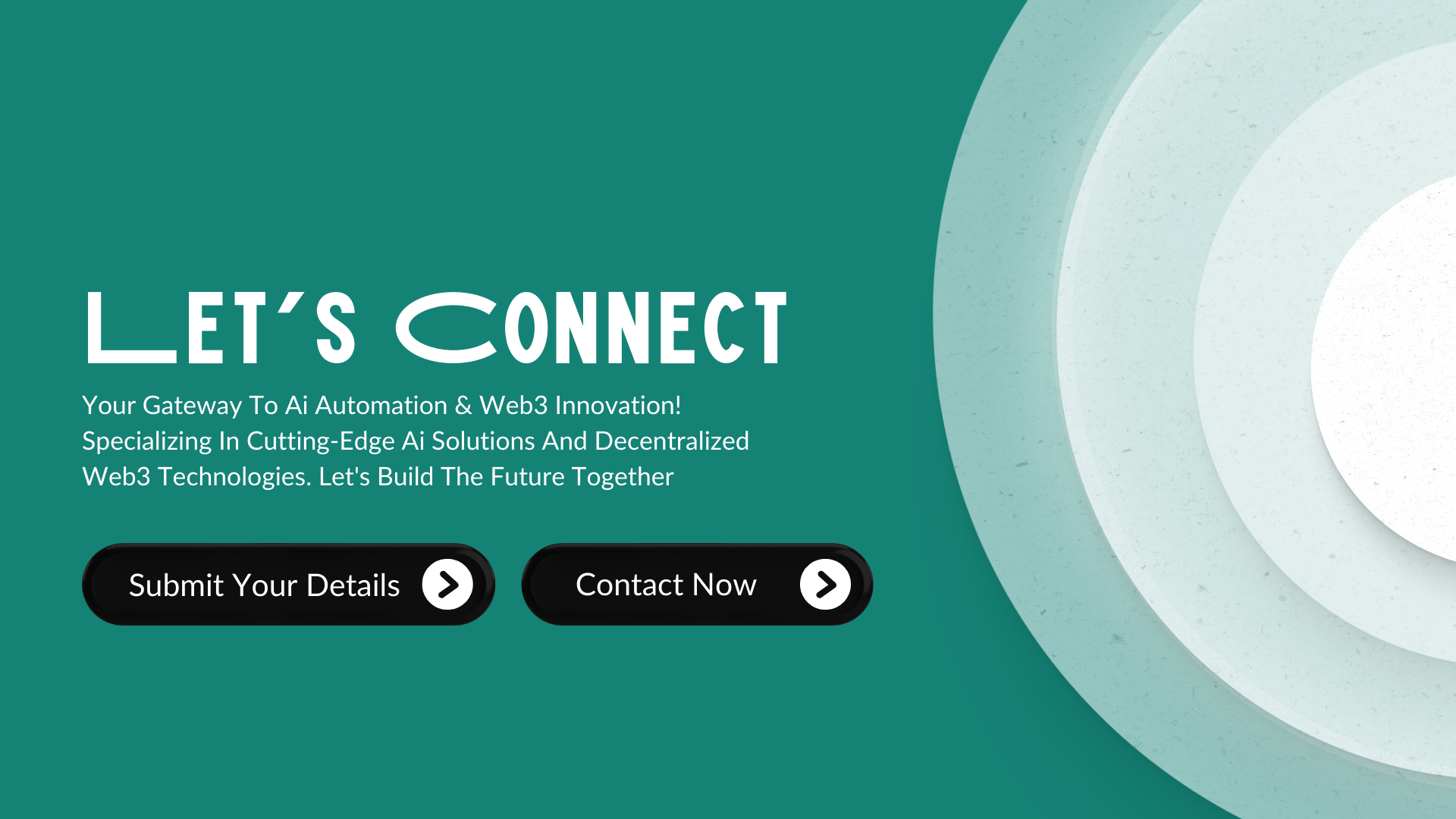